Ralf Wanzenböck
TU Wien
Institute of Materials Chemistry
Tuesday, 29th November 2022,16:00 s.t.
The talk will be given in hybrid mode.
You can either attend in physical presence:
TU Wien, Institute of Applied Physics,
Wiedner Hauptstraße 8-10, 1040 Vienna
Yellow Tower “B”, Seminar Room DB 05 B (5th floor)
Or you join via Zoom:
https://tuwien.zoom.us/j/93979999148?pwd=Q3ZsMXJFVzB3QjBnKzRaZHV6MWNzZz09
Meeting ID: 939 7999 9148 Passcode: nzGD3M0B
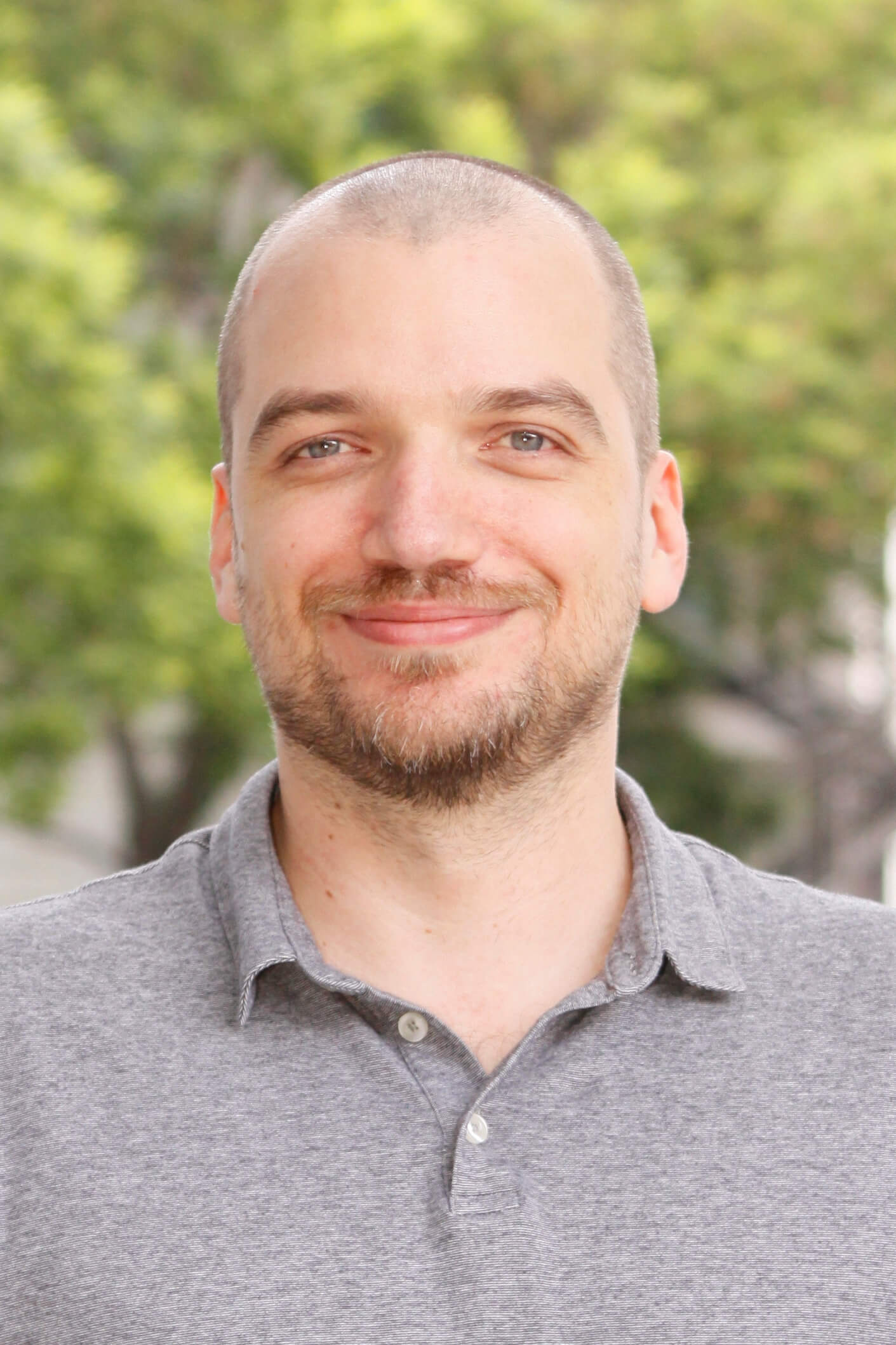
Machine Learning backed Evolutionary Search: Atomic Structure in Surface Reconstructions
Atomic structure determination of surface reconstructions has typically relied on structural models derived from intuition and domain knowledge. While evolutionary strategies combined with first-principles computations based on density functional theory have proven powerful tools for structure searches, the computational cost is prohibitive, even on modern hardware. We facilitate the thorough exploration of potential energy landscapes at reasonable cost by combining the efficient covariance matrix adaptation evolution strategy (CMA-ES) with a neural-network force field (NNFF) utilizing Google JAX with just-in-time compilation and end-to-end automatic differentiation.
The first part of the presentation will cover the methodology used for exploring SrTiO3(110) n×1 overlayer structures and discuss the transferability of the NNFF and the results that were obtained, including known and new low-energy reconstructions. Building on this, an active learning approach that will allow us to investigate more complicated structures, e.g., SrTiO3(110) 2×n surfaces, will be outlined. Finally, an outlook on planned applications of the presented methodology will be given.